As we race towards 2030, artificial intelligence (AI) is now more than just a technological marvel—it’s a colossal economic engine, poised to boost global GDP by up to 14%. That’s an injection of a whopping $15.7 trillion into the world economy, according to PwC research, with retail, financial services, and healthcare standing to realize the most significant gains. But while the promise of AI is dazzling, the reality for many organizations trying to implement these technologies is far from shiny.
Over 85% of AI projects crash before delivering on their potential, draining millions in resources and squandering golden opportunities. Evaluating and implementing an effective AI solution is crucial to avoiding these pitfalls and achieving success.
Why do 85% of AI projects fail?
The importance of strategic AI planning
Many businesses, despite their meticulously pre-planned schedules, struggle to successfully implement their AI projects. In fact, 85% of AI projects fail, according to Gartner’s statistical analysis. Several common challenges contribute to these difficulties, highlighting the critical success factors that need to be addressed to avoid failure.
- One major issue is unrealistic, inflated, and inaccurate expectations. Companies often have an overly optimistic view of what AI can achieve in a short timeframe, leading to disappointment and project failure.
- Another significant problem is the inappropriate labeling of data for training AI components. Accurate and relevant data labeling is crucial for training effective AI models, and any missteps can derail the entire project.
- Another reason is the insufficient support of an organization’s business leaders, as this results in the abandonment of AI projects despite their great potential. A related struggle is change management since AI is not just about using an AI system, but also about redesigning the process where the AI sits.
- Low confidence in the AI model is also a frequent obstacle. If stakeholders don’t trust the AI’s outputs, they are less likely to integrate and utilize the AI solutions, leading to underutilization and failure.
- A persistent challenge is the lack of skilled resources. Given the high demand for AI and data science expertise, many organizations struggle to recruit and retain the necessary talent to advance their projects.
- The ever-growing diversity of data types and formats presents another hurdle. AI systems must be able to process and integrate various data sources seamlessly, which is often a complex and resource-intensive task.
- The lack of quality and quantity of data further complicates AI initiatives. AI models require vast amounts of high-quality data to function effectively, and insufficient data can severely limit a project’s success.
- Underlying technology challenges also play a role. AI projects often require advanced infrastructure and technologies that many organizations may not have readily available, leading to delays and additional costs.
- Another common pitfall is a misalignment of business goals with real-world data. AI models must be designed to solve real business problems, and any disconnect between business objectives and the AI’s capabilities can result in ineffective solutions.
- Lastly, inappropriate futuristic planning can doom AI projects. Forward-thinking strategies are essential, but they must be grounded in realistic assessments of AI’s current capabilities and limitations to avoid unattainable goal-setting.
At the heart of these failures is a fundamental misalignment: companies are all-too-often dazzled by AI’s potential without anchoring their projects to concrete business needs. This misalignment leads to projects being designed more around the capabilities of AI than around the company’s strategic needs.
For AI integration to succeed, companies must first establish a clear, forward-looking business vision that harmonizes with AI’s capabilities. This means a deep-dive into the complexities of AI models to ensure they fit the organization’s specific needs and dynamically allocating resources to keep the projects agile and on track.
Additionally, AI success depends on the necessity of human input and communication. AI professionals must work with subject-matter experts and other teams to vet and prioritize data, improve communication processes, and automate decision-making.
Strategic Approach to AI Implementation: Problem-First Approach
Forget starting with data; start with the problem in the business context. That’s the philosophy behind the ‘Problem First’ approach, which flips traditional AI strategies on their head to significant effect. Instead of wading through data hoping to stumble upon a solution, successful companies identify the business problems needing solutions and then tailor their AI efforts accordingly.
This approach isn’t just theoretical; it’s a practical strategy refined through MindTitan’s extensive experience with EU (and beyond) governmental institutions.
It involves:
- Pinpointing and clearly defining key business challenges.
- Collaborating closely with process experts to shape these challenges into well-defined problems.
- Ensuring AI applications are directly linked to solving these specific issues.
Implementing a machine learning project to address these business issues is at the core of the project’s success and helps generate revenue for the company through the application of AI and machine learning technologies.
Critical Success Criteria for AI Projects
To navigate the AI implementation maze, companies must focus on several critical areas:
Address real business challenges
When embarking on an AI project, it’s essential first clearly to define the business problem you aim to solve. For instance, instead of asking a vague question like “How can we enhance the tax audition system?”, a more precise question such as “Can AI flag issues for tax auditors?” provides a clearer objective and sets the stage for necessary criteria and evaluation metrics.
You need to consider if there’s a clear input that AI could use, the requirements for making such detections, how you can measure the AI’s performance, and what a useful AI output would look like for your business. Incorporating data analysis techniques, such as descriptive statistics, inferential statistics, and visualization, can help in evaluating AI performance effectively.
Assessing the losses incurred due to manual processes will help you understand the baseline from which improvements will be measured. Determine the minimal percentage improvement needed to justify a change in the process. This figure will serve as a crucial benchmark for evaluating the feasibility and success of an AI implementation.
Collaboration between the AI team and the Business team
Partnerships between data scientists and business units is essential to tailor AI solutions to specific business challenges effectively. Framing the problem correctly for the AI project involves discussing the current and desired states of your business processes.
Additionally, working with subject-matter experts is critical to providing the nuanced context that AI tools need but often lack. For example, Fellow Robots, in developing a variety of in-store robots and data collection devices to improve inventory checking, proves that such collaboration simplifies the decisions on data collection and the algorithms for edge processing. Using such meaningful performance metrics as ratios can further aid in evaluating the efficacy and reliability of AI systems.
Prioritize Resource Management and Data Quality
Allocate the right resources and focus intensely on data quality, which forms the backbone of any AI project.
The machine learning partner evaluates whether your business has the necessary data to train relevant ML models and identifies additional frameworks for data collection. To streamline and accelerate this data collection, consider if the data required for AI detection is available and, if not, at what rate it could be collected. Assess the data quality consistency and explore the feasibility of standardizing the data collection process, as high-quality data sources and automated processes help maintain alignment with real-world needs.
Since training an AI model requires a lot of data, determine the speed at which a few thousand samples could be gathered if the process is standardized.
Lastly, consider whether the data collection and labeling tasks can be performed by amateurs or require experts—if extra data is necessary, this will help you estimate expenses.
Choose the right team
Choosing the right AI and ML team is as critical as the technology itself. Whether you’re considering building an in-house team or outsourcing, each route has its merits and challenges. Here’s how you can ensure you’re betting on the right horse when it comes to outsourcing your AI and ML projects.
- Cost Efficiency
Outsourcing reduces costs by eliminating expenses associated with hiring, training, and maintaining a full-time team. Flexible pricing models like per-project or hourly rates further enhance cost-effectiveness. In contrast, in-house teams are difficult to build and load with jobs evenly during a project. - Access to Expertise
Outsourced teams bring specialized knowledge and extensive experience from working across various projects and industries, ensuring the use of the latest AI and ML techniques. For an in-house team, it is either expensive or impossible to hire a specialist for every part of the project. - Scalability and Flexibility
Outsourcing allows for the easy scaling of resources to match project demands without the commitment to long-term employee contracts, offering adaptability to changing project needs. - Faster Project Delivery
Experienced outsourced teams can accelerate project timelines, allowing for quicker market entry and a competitive advantage. - Focus on Core Business
Outsourcing allows companies to concentrate on their primary business areas while experts handle AI and ML tasks, optimizing both technical and strategic business outcomes. - Risk Mitigation
Outsourced providers stay updated with the latest developments and regulatory changes, reducing project risks and ensuring compliance. - Continuous Support and Maintenance
Outsourced teams provide ongoing maintenance and updates, ensuring the AI systems perform optimally over time. - Innovation Leverage
Through working with diverse clients and challenges, outsourced teams bring innovative solutions and perspectives that can significantly improve project outcomes.
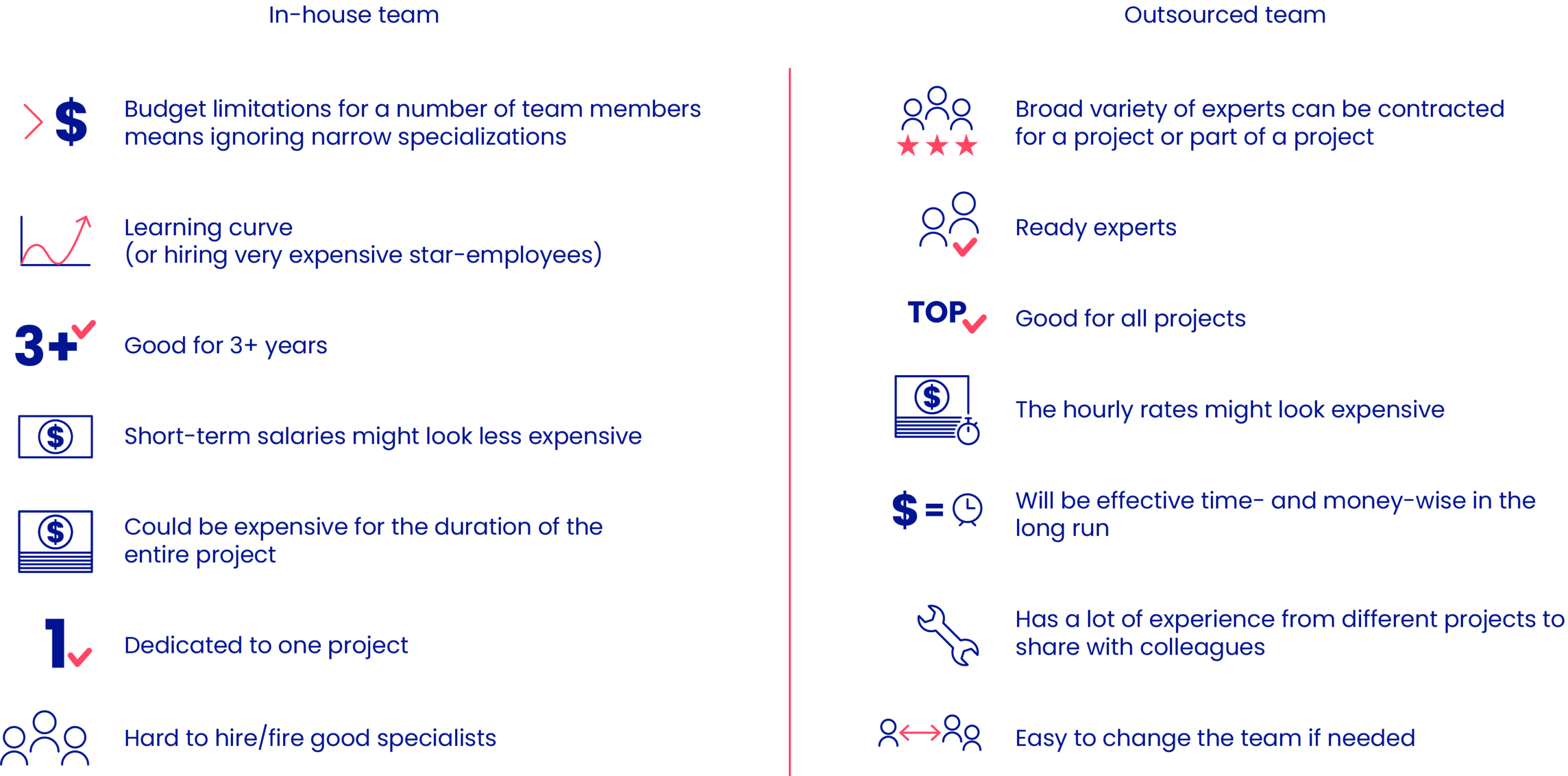
Set Effective Project Management and Operational Integration
Effective AI project management and operational integration are critical for the success of AI initiatives. Adopting structured project management strategies ensures that AI projects are well-organized, with clear milestones, timelines, and deliverables. This approach helps in maintaining focus, resource allocation, and accountability throughout the project lifecycle.
Equally important is the thorough integration and testing of AI models within existing business processes. This step guarantees that the AI solutions are not only technically sound but also seamlessly embedded into the day-to-day operations, thereby maximizing their utility and impact. Rigorous testing ensures that the models perform as expected, reducing the risk of deployment failures and enhancing the overall reliability of the AI system.
It’s also essential that regulations are set up so individuals can protect their data by allowing themselves to opt out of personalized ads or be able to contest decisions made by algorithms, for this contributes towards ensuring an ethical transformation process maintains transparency and accountability simultaneously.
Attention to Education and Demystification
Companies should demystify AI, educating stakeholders on its real capabilities and limitations to foster an environment of trust and innovation. AI isn’t only about technology; it’s about people as well.
Integrating AI into existing processes requires a deft touch, not just in technology adaptation but also in fostering robust team communication and enhancing emotional intelligence within organizations.
Leverage Industry Insights and Expertise
Adopting cross-industry insights can significantly enhance AI applications. Insights from sectors like anomaly detection for banking have proven valuable in refining AI strategies across unrelated fields.
Collaboration with subject-matter experts is crucial for understanding the contextual and nuanced data that AI alone cannot fully interpret. Aligning AI projects with the organization’s strategic priorities ensures that the development of AI solutions is in line with the business’s overarching goals and leads to value-based outcomes.
Best Practices for the Success of AI Projects
To ensure the success of AI projects, organizations should:
- Focus on defining specific business challenges that AI can address.
- Prioritize AI projects based on their potential business impact and the complexity of the required AI solutions.
- Engage cross-functional teams in the planning process to ensure that AI solutions are practical and grounded in real business needs.
- Educate and involve stakeholders to understand AI capabilities and limitations, fostering a culture of innovation and acceptance.
- Integrate AI solutions with existing human-led processes.
Conclusion
Incorporating AI into a business strategy isn’t just about leveraging new technology—it’s about a comprehensive transformation that aligns technical capability with strategic business goals. As we look towards a future where AI is central to business, understanding these dynamics and preparing to tackle them head-on is more crucial than ever.
For organizations ready to take this leap, the journey begins with a clear vision and a strategic approach, ensuring AI not only supports but accelerates their business objectives.