Governing businesses in such complex and technology-intensive fields as Energy & Utilities, entrepreneurs meet the urge to leverage innovations as soon and as much as possible.
Considering the artificial intelligence’s potential to enhance human productivity, 92% of Energy & Utilities companies are either already invested in AI or will do so in the next two years, obtaining competitive advantages. Nearly two-thirds (67%) of energy executives are realizing AI benefits in creating better customer experiences, and more than a half (55% and 53%) in improved decisions making and innovating products and services, PWC reports.
As artificial intelligence tends to be an auspicious technology to play a key role in the future of energy & utilities, let’s dive into AI use cases that bring the most profit.
1. Defect detection and predictive maintenance
One of the challenges that the energy & utilities companies face is detecting suspect pipes/wiring/machines or defects in fault-susceptible processes. Breakages are costly for utilities – in both financial capital and brand value. Defect detection becomes a priority for the field to avoid noticeable losses.
35% of energy executives are realizing AI’s benefits in performing predictive maintenance and automating routine tasks (33%), PWC claims. AI image recognition and computer vision systems allow to cost-effectively process photos and videos of the facilities to alarm employees if any breakage is concerned.
As an example of defect detection, you can check out the computer vision case study of Hepta Airborne and MindTitan collaboration.

Hepta Airborne with the help of drones capturing data assists power grid operators in their power line inspections. A specialized inspection platform called uBird analyzes a vast amount of the visual data from drones by deploying continuous machine learning; it produces a birds-eye view of the grid, and its most critical parts. A detailed report for work crews goes through the AI, where suspicious pictures are flagged for human supervision. This enhancement allowed speeding up the processing up to 15% (ML) now going through 250 km of power line data in 5 minutes and finding 11 more defects per line kilometer on average in comparison to the same process without artificial intelligence.
Moreover, pattern recognition algorithms, processing video streams, or AI image recognition can be used in terms of safety, flagging the violations of adequate dressing for the dangerous operations.

Predictive analytics can alert the operators on the equipment’s health state, enabling proactive actions to prevent health, safety, and environmental damage. Predictive and AI-enhanced maintenance is used by water suppliers as well. AI and machine learning analyze and learn the data patterns that indicate breakage may be imminent so that predictions become more and more accurate with each iteration.
Sensor-rich AI technologies help utility businesses to prevent leaks now. Sophisticate devices are tracking the patterns of liquid flow and usage, establishing the normal stream. When an issue appears, the system either signals to a human about it, pointing to the exact leakage, or shuts off the suppliance, or both. If Energy & Utilities staff is already connected with mobile devices to such a system, it takes much less time to fix the leakage, thus avoiding massive financial and image losses and making their clients happier.
Another example is an AI-based application that enabled operators to pre-empt turbine blades, generators, and gearbox failures on wind turbines whilst optimizing energy production. Cloud-based platforms provide offshore operators with access to advanced analytics software featuring AI algorithms that analyze incoming data for anomalies, ultimately signaling trouble ahead in the monitored equipment.
A beneficial consequence of this AI use case is reducing equipment downtime.
A separate task for utility maintenance teams is to avoid unplanned downtimes that could cost millions of dollars in a single day. For instance, a report by World Economic Forum for the oil & gas industry claims that 92% of shutdowns were caused by unplanned maintenance, costing suppliers an average of $42 million a year to $88 million a year in the worst-case scenarios. By detecting defects and leakages in the early stages, utility companies can reduce the maintenance
2. Transforming the customer experience
The utility suppliers can predict with the help of AI the consumption of water/heating/energy and thus come up with dynamic pricing to offer super low variants when there is excess capacity, for example.
AI provides a highly personalized view of those household interactions with different appliances through sophisticated disaggregation and customer segmentation. Thus, the customer, concerned with the bill size, could adjust and regulate their utility consumption in the most rational way
For instance, artificial intelligence informs a utility that household A has an electric vehicle using a charger between midnight and 6 a.m. each night. Now, utility suppliers have the option to suggest those householders charge their EV earlier when electricity is cheaper.
At the same time, AI gives new opportunities to the marketing department of utility supply companies. Targeting customized and personalized campaigns and loyalty programs. Valuable insights on the customers’ behavior made by AI and then used by a good marketing team can benefit significantly in the long run. For example, using the previous household A, charging an electric vehicle at night, a utility supplier could offer them special discounts or new electric devices and appliances.
3. Forecasting the water and energy consumption
Effective distribution of utility production can be a key to the sustainable growth of supplying companies. According to the USEPA, energy consumption makes up 25-30% of total operation and maintenance (O&M) costs. Artificial intelligence can optimize runtimes of equipment so that they are only used when they are needed. This can be a quick cost-reduction win for many adopters of AI.
In the graph below, you can see the example of a three-zone power system based on data from Great Britain. Supply and consumption have to match in real-time, excess power should be transmitted to the regions with higher demand, and energy transfers between regions are limited by network capacity. Artificial intelligence is invited to create cost-effective strategies for scheduling the power consumption, with the potential savings of the consumer money, and accelerate decarbonization.
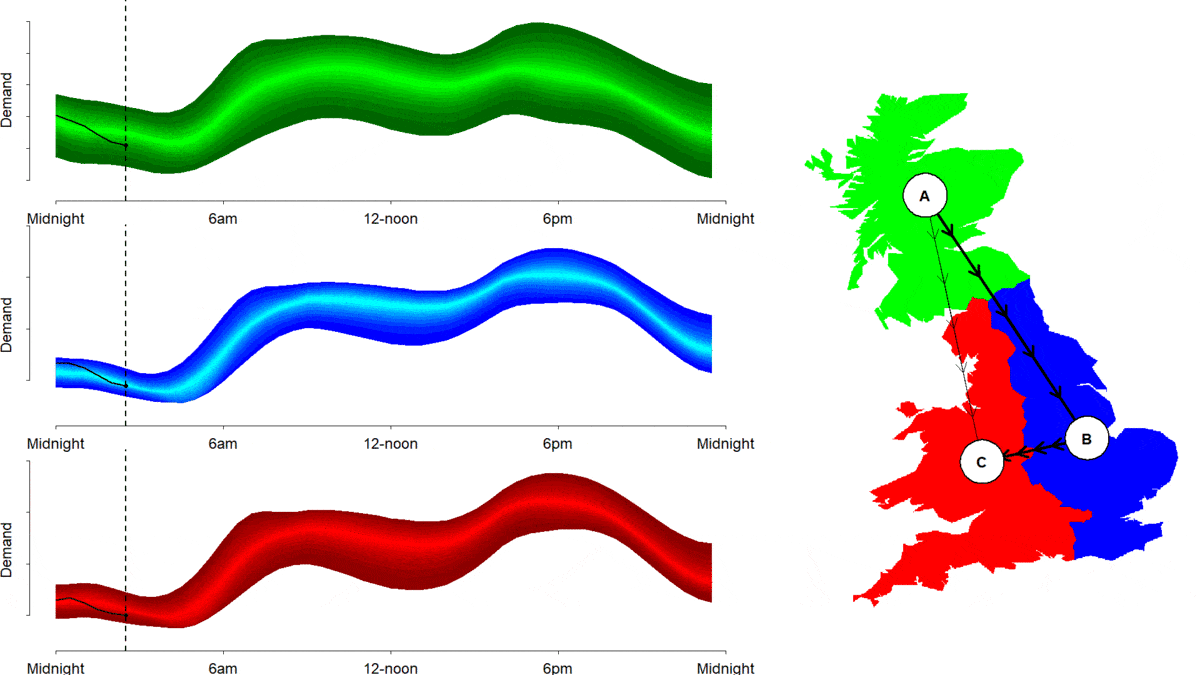
Data analytics tools such as Power BI is used by utilities to predict and plan for future customer demand. Microsoft says its product can “transform reactive decisions to predictive and preventive strategies with enhanced critical equipment and resource management in energy production and distribution channels.”
Microsoft has also developed a means of helping power companies enhance their predictive analytics through Power BI. A suite of business analytics tools that the company says can “improve and transform the way sustainable energy is managed and generate value across energy production, supply, distribution, and consumption by implementing data-driven insights.”
Another side of consumption predictions is defining the load that will fall onto grids or pipes.
Residential energy consumption represents 20% of the overall energy demand, hence showing the importance of reliable and efficient forecasting systems, the latest research claims. In 2017, it was reported that domestic hot water (DHW) contributes to 17% of energy consumption, according to Natural Resources Canada. Therefore, precisely predicting hot water usage is significant for preserving energy. There are algorithms and hybrid artificial intelligence systems that forecast the energy and water consumption, thus predicting the network’s load. Nonetheless, the gap appears in forecasting energy or water demand for abnormal or extreme weather conditions, as well as public holidays, thus it could be your competitive advantage if implemented.
4. Ecologic concerns
A digital twin is an AI model that works as a digital representation of physical equipment or a system. A digital twin assists energy optimization management by providing a simulation environment that can be used to train and test an AI system. These AI models can then be used to monitor and distribute energy and provide forecasts for better service. Algorithms process multiple data sources and visualize them. For example, in a digital twins case study at the Nanyang Technological University, a twin system has been running across 200 campus buildings over five years and managed to save 31% in energy and 9,600 tCO2e.
Despite the economic challenges, many companies are working towards decarbonizing their operations and value chains. BCG studies state that the potential overall impact of applying AI to corporate sustainability amounts to $1.3 trillion to $2.6 trillion in value generated through additional revenues and cost savings by 2030.
AI can also cost-effectively keep the water clean. Meeting effluent compliance standards is a requirement for many organizations – both public and private. AI learns from the unique characteristics you provide, to ensure that effluence standards are met and that compliance fees are avoided.
Manager of General electric explains the steps of AI implementation for utilities.
5. Optimizing energy production and scheduling
31% of energy executives are realizing AI’s benefits provided by analyzing production scheduling scenarios using simulation modeling.
The latest trend in energy efficiency suggests the usage of decentralized microgrids, combining several renewable and non-renewable sources of power, whereas traditionally energy supply chains are centralized and formed around the power plant providing the electricity to the end-users.
Thus, AI technologies can predict the times when energy is effectively produced while the sun is shining or the wind is blowing. Then store the excess of it in their batteries at home because energy is cheap and selling it makes little sense for the supplier.
As well as that, AI can forecast the times of high energy usage, then sell accumulated energy as the prices are higher. The main idea of this AI enhancement is t to maximize micro producers’ profit as well as reduce the expense for end-users.
6. Optimizing logistics network
Certain sectors of the energy sector, such as oil and gas, are demanding in terms of logistics. Its supply chains are sophisticated systems, including several stakeholders and decision-makers, for example, producers, distributors, environmental recycling companies, etc. Artificial intelligence helps coordinate the operations team with the warehouse to ensure the availability of crucial products like the refill tanks. For transmission and distribution processes improvement, AI simulation-based techniques are used a lot often alongside IoT tech.
For example, AI can help process a vast amount of data from customers, producers, and much more, like traffic data or truck GPS locations, to support fleet optimization, convenient planning, better route selection, etc. Moreover, it helps predict and refine market prices for gas and electricity.
In brief, machine learning assists energy companies in handling shipping operations for replacing assets, risk hedging, and improving delivery times as well as reducing overall costs.
7. Enhancing inventory management
When inventory lags demand, companies suffer losses. AI helps ramp up efficiencies in network and infrastructure planning, allowing merchandisers to become more proactive. For instance, New York is planning to transition to clean energy by 2040, thus there are questions like how many solar panels or wind turbines to install, and how much energy storage capacity to build? The answer is hidden somewhere in the data, so it is the task of AI.
Renewable sources of energy such as offshore wind farm projects face challenges of high price and delays because of weather or resource limitations while building the facilities. Moreover, obstacles can pop up because of law and government restrictions, and ecological and biological considerations. All this leads to the vital necessity of creating a powerful and reliable project planning and scheduling AI models that process all of these influential factors to build an offshore wind farm in time and run it efficiently.
As energy & utility companies (including ones that work with renewable energy sources) gain more and more visibility into the demand patterns, they can plan inventory changes, like with new driving habits, for example adjusting the number of charging points and/or offering clients to use nearby not occupied recharging points for electric vehicles. This leads to happier customers and lower operational costs.
8. AI-Powered increasing the efficiency of Energy Storage
According to EPRI, current new-energy storage systems “are typically 4-hour duration or less, corresponding to peaking capacity and ancillary services needs. However, in the coming years as storage is deployed to replace higher capacity factor conventional generation, absorb longer periods of renewable overgeneration, and support resilience during severe weather events there is a potential need for longer duration storage.”
Material research, prototyping, testing, validation, and lifecycle assessment that used to take a lot of time in the labs can now be done in a virtual laboratory. Thus, it can speed up the research and development of new materials. Because of the rapid storage and release of energy from renewable sources, the requirements of high charge and discharge rates and low cost are becoming increasingly important. Thus, AI and ML are used to predict new battery material discovery and establish a new understanding of material behavior.
Conclusion
Easy to notice that we live in a time when two separate trillion-dollar industries — the energy industry and the data and information industry — are now intersecting in a way they never have before. Machine learning technologies have the potency to pivot the utility industry and bring us to a better world, enhancing safety, optimizing water and energy usage, and caring for the environment and user experience.
Thus, AI implementation is not easy, but for smooth grasping, you can imagine it as several steps of an execution plan for an AI project: define a problem to solve with artificial intelligence and set up business goals, set up a team for an AI project, explore your data, develop and test the AI model, then deploy it and constantly improve your AI system.
The good news is that often utility companies already have the very first and most important part of the AI: data. So the next step to the enhanced processes is searching for the right use case, then cleaning this data and getting profit with a good AI model.
While there are many off-the-shelf AI solutions and platforms available on the market, these offers may not be perfectly optimized for your needs, may solve only a part of the problem, or might cause you to deal with symptoms/problems instead of the actual root of the problem. Contrarily, custom AI solutions will yield the most accurate results and solve the business problem. Note: it could happen only with a team of high-profile AI and ML specialists.