In a corporate world alive with constant change, AI stands as the new frontier, transforming every tick of the business clock into an opportunity for innovation. As we stand in 2024, AI has ceased to be a futuristic promise—it is now the core engine of the global economy, driving enterprises towards a horizon of untapped potential.
It’s time to cast aside the traditional playbook. The AI revolution isn’t coming—it’s already here, and it’s rewriting the rules of engagement across healthcare, manufacturing, and beyond. This is where strategy meets science, where your vision harnesses the precision of AI to catapult your organization to the forefront of innovation.
Stand at the helm of this transformation, and lead your organization into an era where operational renaissance is a daily reality. With AI, the future of industry leadership is yours to define. Let’s journey through this evolution together, where the outcomes aren’t just beneficial—they’re revolutionary.
The Essence of AI Transformation
From highly competent virtual assistants handling various tasks or production floor automation speeding up processes significantly while reducing errors, adopting effective AI solutions means advantages for businesses.
But what does this pivot to Artificial Intelligence precisely mean? It not only allows access to sophisticated technologies – such as automated systems and creative applications – but also challenges organizations to go through structural changes and enhancements due to working with AI solutions.
Defining AI Transformation
AI Transformation refers to the process by which businesses and organizations integrate Artificial Intelligence into their operations to improve efficiency, enhance performance, and create new opportunities. Additionally as noted above, it’s a comprehensive change that affects all aspects of the organization, including its processes, data management, skill sets, and even its culture.
Here are the key components of AI Transformation:
Strategic Vision and Leadership:
- Developing a clear vision of how AI can benefit the organization and communicate this effectively.
- Ensuring alignment of strategic planning for AI with the organization’s overall business goals.
Cultural Change:
- Creating a culture that embraces change, innovation, and continuous learning.
- Encouraging collaboration between AI experts and domain specialists within the organization.
Data Strategy:
- Understanding the importance of data as a foundational element for AI.
- Investing in the right infrastructure and practices for data collection, processing, and analysis.
Technology Infrastructure:
- Building or acquiring the necessary technological tools and platforms that enable AI.
- Ensuring interoperability and scalability of AI technologies within existing IT systems.
Talent:
- Upskilling existing staff to work effectively with AI.
- Work with outside AI experts to get timely results.
- Attracting or developing new talent with expertise in AI, machine learning, and data science.
Governance and Risks:
- Developing guidelines and policies for the responsible use of AI.
- Ensuring compliance with relevant laws and regulations.
- Considering the end-customer and direct user reactions.
Use Case Development:
- Identifying and prioritizing potential AI use cases that prove value to the organization.
- Starting with pilot projects that can provide quick wins and exploit value.
Scaling AI Initiatives:
- Once successful pilot projects are complete, organizations need to scale these solutions across the organization to maximize impact.
Organizations worldwide are embarking on AI transformation, considered the next stage of digital transformation. Businesses that adopt this form of change will find themselves experiencing increased efficiency and innovative opportunities like a butterfly emerging from its cocoon.
The Impact of AI Transformation
The increased use of AI is impacting numerous industries, from finance to transportation. AI integration has become even more popular now, as seen with advances within the automotive, high-tech communication, energy, and financial sectors, allowing companies access to a range of benefits such as listed below.
Customer Experience Enhancement:
AI tools can bridge the gap between customer expectations and business responsiveness. From chatbots to personalized systems, AI can deliver immediate, tailored interactions, increasing customer satisfaction and operational efficiency.
Error Reduction:
AI-driven processes can eliminate the ‘human error’ factor, allowing machines to perform repetitive tasks with precision.
Automation Impact:
AI not only automates tasks but also boosts overall process efficiency—such as in manufacturing where it can improve yield and lower costs. It liberates employees from mundane tasks, allowing them to engage in more innovative and strategic activities.
Complex Problem Solving:
AI excels at addressing complex issues, offering scalable solutions and freeing up human intelligence for critical thinking and problem-solving.
Operational Efficiency:
By automating repetitive tasks and minimizing errors, AI contributes to a significant increase in operational efficiency, with potential productivity gains of up to 54%.
Better Decisions:
AI enhances decision-making by processing large datasets efficiently, providing trend analysis, and forecasting outcomes, all without the biases of human emotion.
By applying these technologies, organizations have the opportunity to develop their internal strengths, increase their decision-making capabilities and improve product performance to be competitive on a global scale.
Steps to Implement AI Transformation
Now, it’s time to consider the steps organizations can take to ensure a successful AI transformation. An AI strategy will help smooth this journey and effectively bring about an organizational switchover from traditional ways of doing things towards utilizing technology-oriented approaches.
The most beneficial actions for implementing an AI transformation process address its key components: planning and preparation, testing, implementation, and integration, as well as training teams on new technologies associated with the transition.
Phase 1. Business Understanding
Phase 1, Business Understanding, as mentioned above is to discern and comprehend the business problems amenable to an AI application. This demands a significant understanding of the underlying challenges, setting specific objectives, and AI success criteria to ensure that any AI deployment is well-founded.
Identifying business issues, a critical first step, involves a methodical approach to discerning challenges that may benefit from AI solutions. This process begins with engaging experts who have a deep understanding of corporate operations to isolate specific problems. Viability queries follow, assessing whether a task can be completed efficiently by a person with adequate knowledge or whether it fits into a predictable pattern—a positive indication of a task’s AI suitability.
Key to this is the identification of AI suitability standards, where tasks ideal for AI are those that are repetitive, amenable to learning loops, and those that require minimal interaction with a complex physical environment. Standard, routine tasks that involve many operatives, particularly those that are analytically demanding or involve complex data relationships, such as auditing social media posts or predicting traffic patterns, are prime candidates for AI integration.
Phase 2. Preparation
Phase 2, Preparation, aims to ascertain if the AI can fulfill the intended business goals via a proof of concept (PoC).The key undertakings include:
- Amassing, prepping, and marking data to ensure its excellence, volume, and diversity for efficacious model training.
- Crafting data conduits that enable data movement and alteration from source to end-use.
- Formulating and appraising PoC to check feasibility and practicability and to delineate the parameters and needs for an Minimum Viable Product MVP.
- Strategizing the pilot, minding users, tasks, timelines, and budget, as well as depicting the successful MVP.
Phase 3. Pilot
The next Phase 3, Pilot, is dedicated to constructing an MVP that’s feature-rich enough for initial user feedback, pivotal for validating the potential for full-scale production. Through conducting experiments or tests on smaller scales, organizations can evaluate if a project idea has potential and identify possible risks. With feedback from stakeholders, decisions about launching an actual full-scale implementation will be informed.
Primary tasks include:
- Assembling an MVP to allow end-user interaction with AI outcomes and to garner live feedback.
- Examining the MVP in actual settings to affirm the solution and collect market insights.
- Assessing the model with pre-established metrics to verify its contribution to business value.
- Refining the model grounded on real-world feedback and trials to rectify any issues.
Phase 4. Production
Phase 4, Production, involves transitioning from a successful pilot to a full-scale application. This is done through the establishment of Machine Learning Operations (MLOps) practices, ensuring the ML models are efficiently integrated into production environments and the application is ready for real users. The development of a comprehensive reporting system is crucial to monitor performance and outcomes. The AI system will continue to evolve and improve over time, much like any other IT system, with maintenance and iterative updates based on new data and potentially shifting business goals.
Phase 5. Scaling
Phase 5, Scaling, focuses on the expansion of the AI application’s capacity to ensure it can handle increased loads without downtime, which involves attention to high availability, load balancing, and fault tolerance. Scaling can also mean extending the AI solution to additional business areas or customer segments, but this requires time to succeed. The result is a widely deployed AI project that delivers significant business value and benefits.
After the AI feature or tool is up and running, it’s important to circle back to your original performance indicators. Review your key performance indicators (KPIs) and return on investment (ROI) metrics to assess whether the AI implementation is delivering the intended benefits and meeting organizational needs. This post-implementation analysis is vital for validating the business impact of the AI solution and guiding any necessary adjustments to maximize its effectiveness.
Overcoming Obstacles in AI Transformation
Navigating the journey of Artificial Intelligence (AI) transformation presents a number of challenges, such as a lack of qualified personnel and resistance to alteration. Businesses need to face these impediments without hesitating in order to raise the AI adoption rate. Here is how organizations can overcome their roadblocks:
Tackling Talent Shortages
Outsourcing AI development should be the first option for most organizations considering AI projects. This is primarily because building in-house AI capabilities is a lengthy and complex process, and AI projects are typically developed in a relatively short period and have a high failure rate. Over 50% of AI projects fail when managed internally, making outsourcing a logical and strategic decision.
Reasons to opt for outsourcing machine learning team:
- Expertise on Demand: Outsourcing machine learning team provides immediate access to top-tier talent and cutting-edge technologies, allowing companies to leapfrog the lengthy and costly process of building these capabilities from scratch.
- Cost-Effectiveness: It is more economical in the short term compared to the high costs associated with hiring, training, and retaining specialized in-house teams.
- Scalability and Flexibility: Outsourcing offers the flexibility to scale AI efforts up or down based on business needs without the ongoing commitment to an internal infrastructure.
Considerations for an In-House AI team:
While having control and the ability to tailor AI solutions are significant advantages of in-house development, the financial and logistical challenges often outweigh these benefits in the long run. The necessity to assemble a diverse team of analysts, data scientists, developers, and other specialists—each of whom plays a crucial role at different stages of an AI project—can be a formidable obstacle, especially for organizations without a strong initial foundation in AI and machine learning.
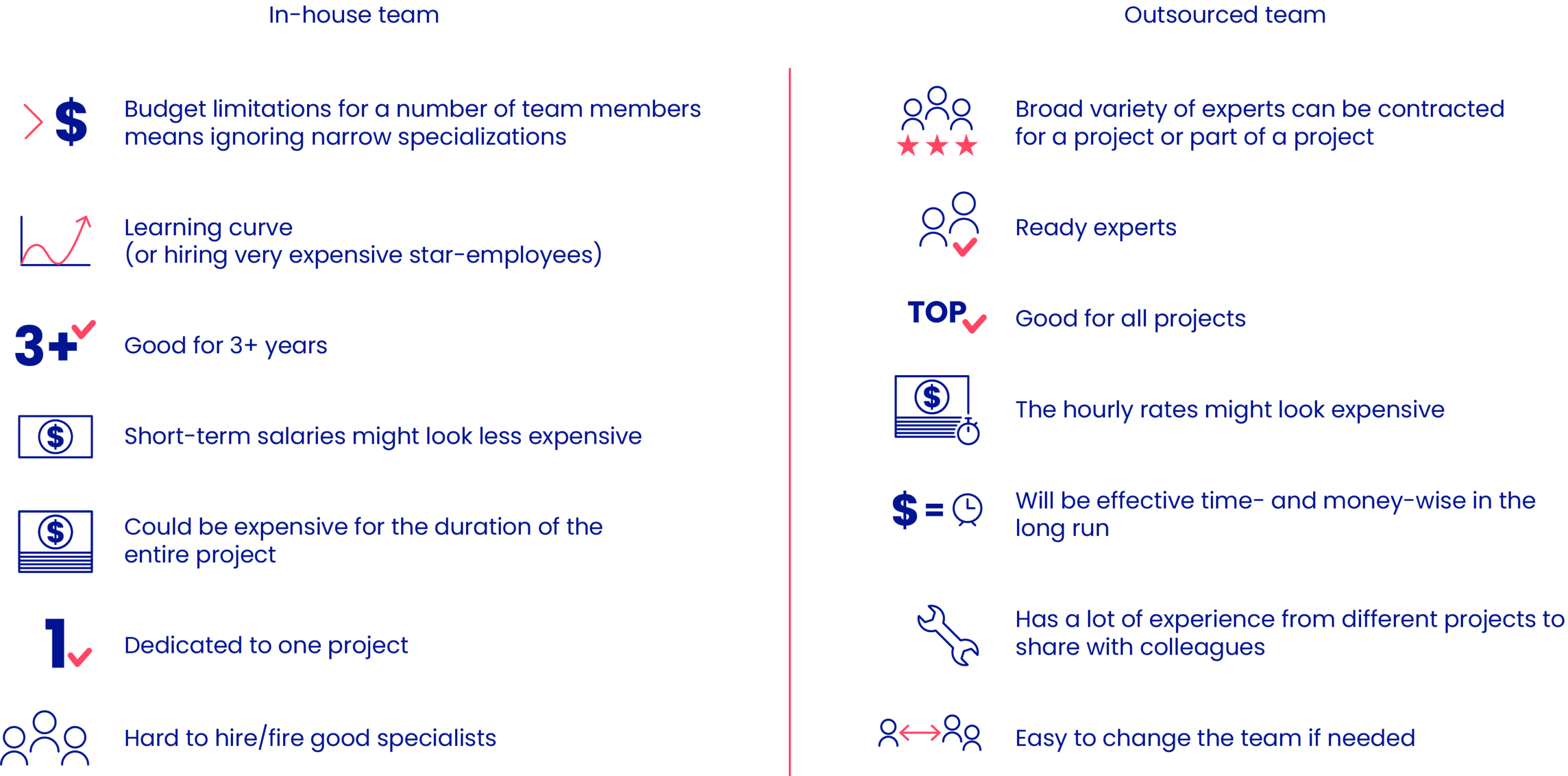
Given these dynamics, businesses should critically assess their long-term strategy, operational capacity, and financial constraints to determine whether outsourcing or in-house development is more aligned with their overall objectives. For most, outsourcing emerges as the more pragmatic approach to circumvent the common pitfalls of AI projects and leverage the benefits of artificial intelligence swiftly and effectively.
Managing Resistance to Change
Organizations must address the common hesitations to AI transformation that arise when it comes to changes in business processes, structure, and employee roles. To move a company forward with this process successfully, all stakeholders should be included in decisions made as well as foster an environment of open dialogue and innovation throughout the company. Through these methods, businesses can create a culture conducive to embracing AI change for growth opportunities, one focused on progress rather than resistance.
It’s also essential that regulations are set up so individuals can protect their data by allowing themselves to opt out of personalized ads or be able to contest decisions made by algorithms, for this contributes towards ensuring an ethical transformation process maintains transparency and accountability simultaneously.
Keys to a Successful AI Transformation Strategy
The key to achieving a fruitful AI transformation is constructing an effective foundation. Think of it like building a sturdy bridge, connecting the current state of your business with what it could be in an AI-driven future. What are the essential pieces for having a prosperous plan for transitioning into Artificial Intelligence?
AI Team
For any successful AI project, a robust team should extend beyond a couple of data scientists to include various specialized roles.
The roles in AI project execution can generally be categorized into three groups: the problem owner side, the AI solution side, and the connectors. On the problem owner side, we have the problem owner and tech support specialists who define the issue and requirements. The AI solution side comprises roles like data scientists, data engineers, machine learning engineers, analysts, and software engineers, all focused on crafting the AI solution. Bridging these two domains is the project manager, who ensures cohesion and facilitates communication between the problem owner and the solution team.
(You can read more about AI and machine learning team in our blog)
Successful AI projects require a balanced team from the start, especially business-oriented members who ensure that the project is addressing the right problem. It’s crucial to understand that AI development is iterative: initial implementation and data gathering lead to new cycles of refinement and possibly evolving business objectives.
So, first and foremost, you must have a strong awareness of how to execute an AI project securely and correctly from the problem owner (aka business-oriented) side.
Establishing Clear Business Goals
Business goals provide a beacon for companies on their AI transformation journey. Establishing what you want to achieve, assessing the present state of affairs, and creating strategies that link with overarching objectives helps guarantee your AI initiatives are properly aligned with corporate plans.
For example, when used strategically within the retail industry, Artificial Intelligence can improve both efficiency and customer experience by ensuring allocated resources are utilized in the most effective way and tasks are rated by importance accordingly.
In other words, having clear business aims allows companies to choose the right processes to transform while tracking progress all along the way.
Building a Robust Infrastructure
Building a dependable infrastructure for AI transformation is like laying the groundwork for constructing a skyscraper. It requires an IT system that can store, process and scrutinize high amounts of data to provide your organization with the technology needed to achieve its AI objectives. With a robust foundation in place, enterprises are better equipped to:
- store massive datasets;
- handle processing efficiently;
- accurately analyze their findings while being able to customize capabilities as necessary depending on requirements and needs.
Having this strong base allows companies embarking upon their AI journey to have a better chance at success due to reliable connectivity when they need it most, along with adaptability so any changes won’t be too difficult or costly.
Allocating Adequate Resources
Organizations must make sure their AI projects are resourced adequately if they want to achieve success. This means investing in appropriate technology and personnel, such as data engineers, as well as providing comprehensive training around AI and reserving resources for running pilot tests on the initiatives taken. With adequate funds and other substantial recourse dedicated to the initiative, such as a well-staffed team in place, businesses can expedite their AI transformation journey effectively.
Providing sufficient resources is necessary when launching an organization’s endeavors to integrate more automation capabilities. It’s like fueling up a rocket before sending it out of Earth’s atmosphere– without enough fuel or power, you won’t get far!
Best Practices for AI Transformation
Enterprises aiming to achieve a profitable AI transformation should adhere to established best practices as they go through the process. This can provide guidance and aid them in attaining their desired results.
Aligning AI Initiatives with Business Goals
Organizations can ensure their AI initiatives are directed towards producing the required outcomes by connecting them with predetermined business objectives. For instance, Artificial Intelligence has been employed in retail to enhance customer service and cut down expenses simultaneously. By carefully aligning AI technologies with its ultimate goals, a business will be able to focus its energies and allocate resources on projects that have maximum impact.
Fostering Collaboration and Communication
Businesses that want to embrace AI transformation and drive innovation can do so by creating an environment characterized by open dialogue, feedback and collaboration. Involving all stakeholders in the decision-making process of this transition is akin to conducting a symphony: everyone’s part combining harmoniously. This ensures that there is alignment between them on their respective journeys towards change.
Summary
In short, the AI transformation of a modern enterprise is a journey filled with numerous possibilities and difficulties. By comprehending the heart of AI conversion, applying basic aspects for an effective strategy, and utilizing optimal practices, businesses can move boldly on this pathway and gain momentum toward desired objectives.
As we look into the future of business life worldwide, a future defined by artificial intelligence (AI), companies that need to stay competitive have no choice but to adopt the transformative power of such AI technology. Are you ready to implement the transforming strength provided by Artifical Intelligence, leading your organization forward and reaching a new level of innovation & success?